Weighted vs Unweighted average for azimuthal integration#
The aim of this tutorial is to investigate the ability to preform unweighted averages during azimuthal integration and validate the result for intensity and uncertainties propagation.
[1]:
%matplotlib inline
# use `widget` instead of `inline` for better user-exeperience. `inline` allows to store plots into notebooks.
import time
import numpy
from matplotlib.pyplot import subplots
from scipy.stats import chi2 as chi2_dist
import fabio
import pyFAI
from pyFAI.gui import jupyter
from pyFAI.test.utilstest import UtilsTest
from pyFAI.utils.mathutil import rwp
t0 = time.perf_counter()
[2]:
img = fabio.open(UtilsTest.getimage("Pilatus1M.edf")).data
ai = pyFAI.load(UtilsTest.getimage("Pilatus1M.poni"))
print(ai)
jupyter.display(img)
Detector Pilatus 1M PixelSize= 172µm, 172µm BottomRight (3)
Wavelength= 1.000000e-10 m
SampleDetDist= 1.583231e+00 m PONI= 3.341702e-02, 4.122778e-02 m rot1=0.006487 rot2=0.007558 rot3=0.000000 rad
DirectBeamDist= 1583.310 mm Center: x=179.981, y=263.859 pix Tilt= 0.571° tiltPlanRotation= 130.640° 𝛌= 1.000Å
[2]:
<Axes: >
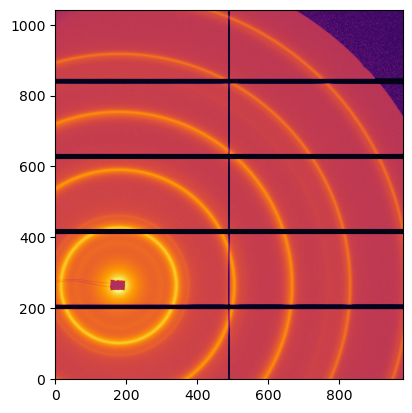
[3]:
method = pyFAI.method_registry.IntegrationMethod.parse(("no", "csr", "cython"), dim=1)
weighted = method
unweighted = method.unweighted
[4]:
#Note
weighted.weighted_average, unweighted.weighted_average, weighted == unweighted
[4]:
(True, False, True)
[5]:
res_w = ai.integrate1d(img, 1000, method=weighted, error_model="poisson", polarization_factor=0.99)
res_u = ai.integrate1d(img, 1000, method=unweighted, error_model="poisson", polarization_factor=0.99)
rwp(res_u, res_w)
[5]:
np.float64(0.0026848043468634406)
[6]:
ax = jupyter.plot1d(res_w, label="weighted")
ax = jupyter.plot1d(res_u, label="unweighted", ax=ax)
ax.plot(res_w.radial, abs(res_w.intensity-res_u.intensity), label="delta")
ax.legend()
ax.set_yscale("log")
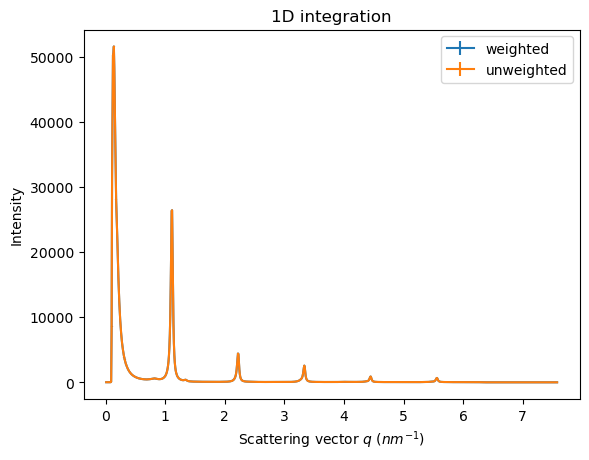
About statstics#
Work on a dataset with 1000 frames in a WAXS like configuration
[7]:
ai_init = {"dist":0.1,
"poni1":0.1,
"poni2":0.1,
"rot1":0.0,
"rot2":0.0,
"rot3":0.0,
"detector": "Pilatus1M",
"wavelength":1e-10}
ai = pyFAI.load(ai_init)
unit = pyFAI.units.to_unit("q_A^-1")
detector = ai.detector
npt = 1000
nimg = 1000
wl = 1e-10
I0 = 1e4
polarization = 0.99
kwargs = {"npt":npt,
"polarization_factor": polarization,
"safe":False,
"error_model": pyFAI.containers.ErrorModel.POISSON}
print(ai)
Detector Pilatus 1M PixelSize= 172µm, 172µm BottomRight (3)
Wavelength= 1.000000e-10 m
SampleDetDist= 1.000000e-01 m PONI= 1.000000e-01, 1.000000e-01 m rot1=0.000000 rot2=0.000000 rot3=0.000000 rad
DirectBeamDist= 100.000 mm Center: x=581.395, y=581.395 pix Tilt= 0.000° tiltPlanRotation= 0.000° 𝛌= 1.000Å
[8]:
# Generation of a "SAXS-like" curve with the shape of a lorentzian curve
flat = numpy.random.random(detector.shape) + 0.5
[9]:
qmax = ai.integrate1d(flat, method=method, **kwargs).radial.max()
q = numpy.linspace(0, ai.array_from_unit(unit="q_A^-1").max(), npt)
I = I0/(1+q**2)
jupyter.plot1d((q,I))
pass
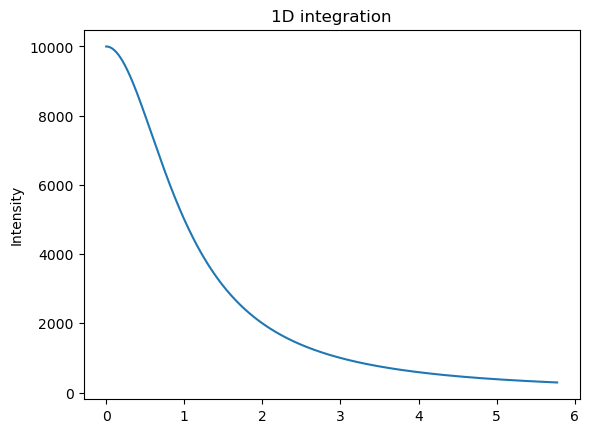
[10]:
#Reconstruction of diffusion image:
img_theo = ai.calcfrom1d(q, I, dim1_unit=unit,
correctSolidAngle=True,
flat=flat,
polarization_factor=polarization,
mask=ai.detector.mask)
kwargs["flat"] = flat
jupyter.display(img_theo, label="Diffusion image")
pass
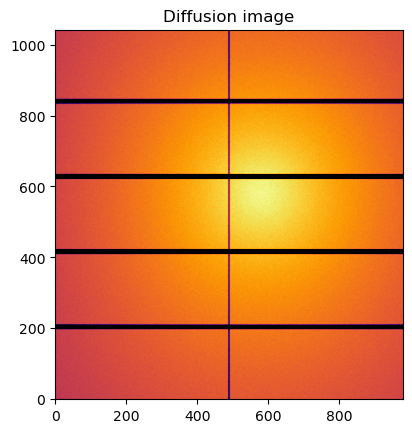
[11]:
res_theo = ai.integrate1d(img_theo, method=method, **kwargs)
jupyter.plot1d(res_theo)
[11]:
<Axes: title={'center': '1D integration'}, xlabel='Scattering vector $q$ ($nm^{-1}$)', ylabel='Intensity'>
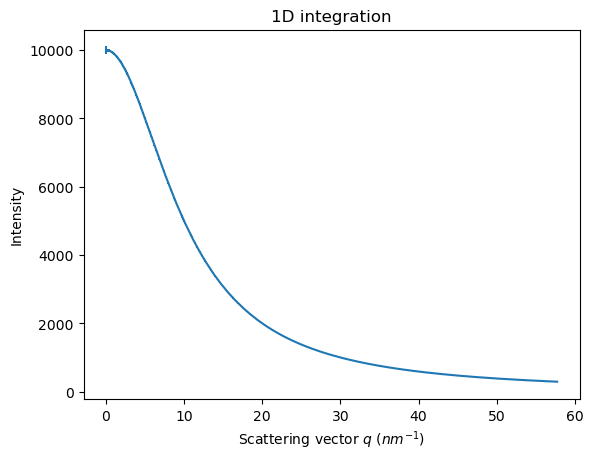
[12]:
%%time
if "dataset" not in dir():
dataset = numpy.random.poisson(img_theo, (nimg,) + img_theo.shape)
# else avoid wasting time
print(dataset.nbytes/(1<<20), "MBytes", dataset.shape)
7806.266784667969 MBytes (1000, 1043, 981)
CPU times: user 54.5 s, sys: 46 s, total: 1min 40s
Wall time: 1min 41s
[13]:
res_weighted = ai.integrate1d(dataset[0], method=weighted, **kwargs)
res_unweighted = ai.integrate1d(dataset[0], method=unweighted, **kwargs)
ax = jupyter.plot1d(res_theo, label="theo")
ax = jupyter.plot1d(res_weighted, label="weighted", ax=ax)
ax = jupyter.plot1d(res_unweighted, label="unweighted", ax=ax)
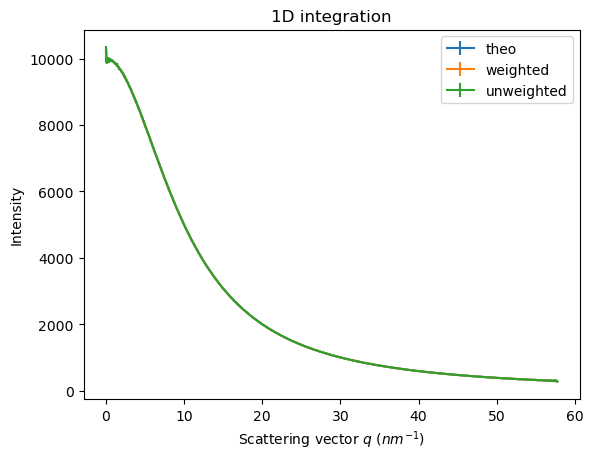
[14]:
fix,ax = subplots(2, figsize=(12,4))
ax[0].plot(res_theo.radial, res_theo.intensity, label="theo")
ax[1].plot(res_theo.radial, res_theo.sigma, label="theo")
ax[0].plot(res_weighted.radial, res_weighted.intensity, "3", label="weighted")
ax[1].plot(res_weighted.radial, res_weighted.sigma, "3",label="weighted")
ax[0].plot(res_unweighted.radial, res_unweighted.intensity, "4", label="unweighted")
ax[1].plot(res_unweighted.radial, res_unweighted.sigma, "4", label="unweighted")
ax[0].set_ylabel("Intensities")
ax[1].set_ylabel("Uncertainties")
_ = ax[0].legend()
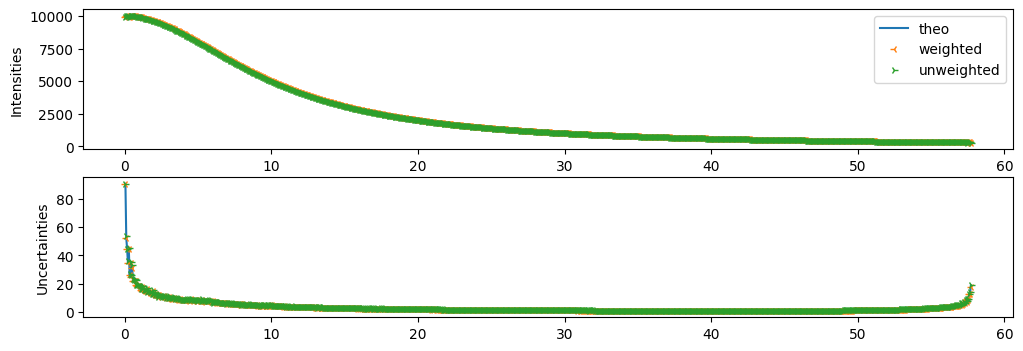
[15]:
print("Number of paires of images: ", nimg*(nimg-1)//2)
Number of paires of images: 499500
[16]:
def chi2_curves(res1, res2):
"""Calculate the Chi² value for a pair of integrated data"""
I = res1.intensity
J = res2.intensity
l = len(I)
assert len(J) == l
sigma_I = res1.sigma
sigma_J = res2.sigma
return ((I-J)**2/(sigma_I**2+sigma_J**2)).sum()/(l-1)
[17]:
def plot_distribution(ai, kwargs, nbins=100, method=method, ax=None):
ai.reset()
results = []
c2 = []
integrate = ai.integrate1d
for i in range(nimg):
data = dataset[i, :, :]
r = integrate(data, method=method, **kwargs)
results.append(r)
for j in results[:i]:
c2.append(chi2_curves(r, j))
c2 = numpy.array(c2)
if ax is None:
fig, ax = subplots()
h,b,_ = ax.hist(c2, nbins, label="Measured histogram")
y_sim = chi2_dist.pdf(b*(npt-1), npt)
y_sim *= h.sum()/y_sim.sum()
ax.plot(b, y_sim, label=r"$\chi^{2}$ distribution")
ax.set_title(f"Integrated curves with {integrate.__name__}")
ax.set_xlabel("$\chi^{2}$ values (histogrammed)")
ax.set_ylabel("Number of occurences")
ax.legend()
return ax
[18]:
fig,ax = subplots(1,2, figsize=(12,4))
a=plot_distribution(ai, kwargs, method=unweighted, ax=ax[0])
a.set_title("Unweighted")
a=plot_distribution(ai, kwargs, method=weighted, ax=ax[1])
a.set_title("Weighted")
[18]:
Text(0.5, 1.0, 'Weighted')
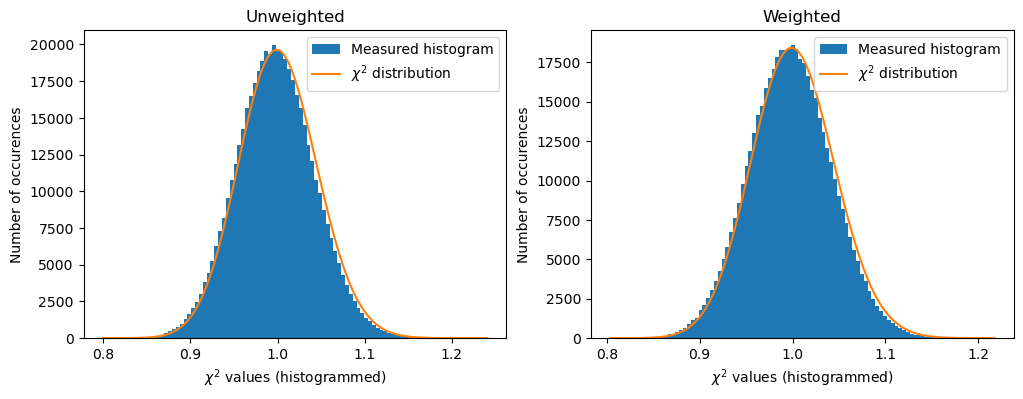
[19]:
print(rwp(ai.integrate1d(dataset[100], method=weighted, **kwargs),
ai.integrate1d(dataset[100], method=unweighted, **kwargs)))
0.05028434923207519
[20]:
print(f"Total run-time: {time.perf_counter()-t0:.3f}s")
Total run-time: 213.239s
Conclusion#
The two algorithms provide similar results but not strictly the same. The difference is largely beyond the numerical noise since the Rwp between two results is in the range of a few percent. Their performances for the speed is also equivalent. Their results are different but on a statistical point of view, it is difficult to distinguish them.
To me (J. Kieffer, author of pyFAI), the question of the best algorithm remains open.
[ ]: